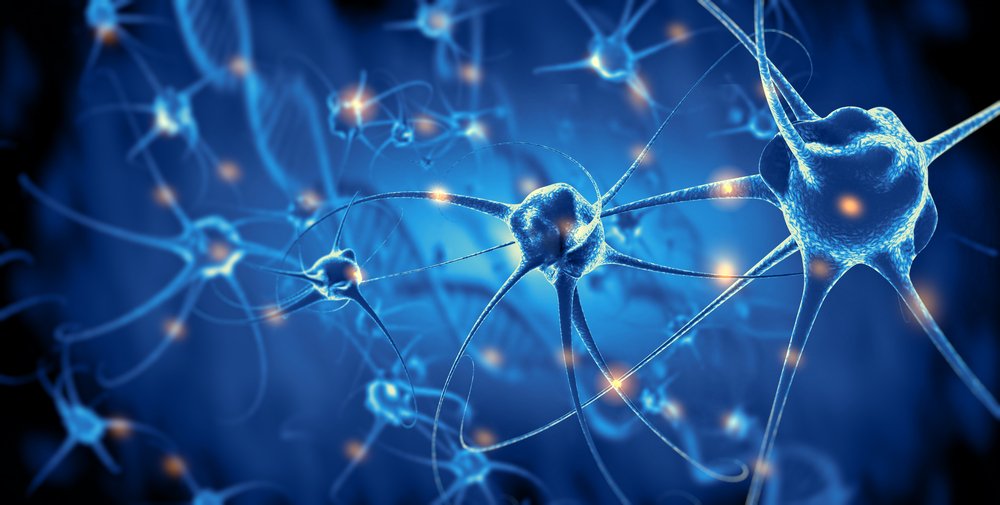
A NEAR AND DISTANT PAST
The Artificial Neuron, referred to in English as Percetron, translated into Italian now as Percetrone or Percettrone has not recent origins; based with the times of Computer Science . Its theoretical basis goes back to 1946 and draws origins from multiple disciplines: Biology, Mathematics, Physics, Chemistry.
The perceptron arose from an early draft of an artificial neuron theorized by Warren Mc Culloch and Walter Pitts.
The idea of the artificial neuron was later taken up by Frank Rosenblatt who developed an electromechanical machine consisting of potentiometers, motors, and photocells that would be intended to recognize simple geometric shapes.
Perceptron was a simple binary linear classifier and thus was able to effectively learn the rule needed to recognize two different, linearly separable input classes.
Frank Rosenblatt, in addition to physically implementing it, also studied a simple algorithm for training.
The idea of the artificial neuron was later taken up by Frank Rosenblatt who developed an electromechanical machine consisting of potentiometers, motors, and photocells that would be intended to recognize simple geometric shapes.
The perceptron was a simple binary linear classifier and thus was able to effectively learn the rule needed to recognize two different, linearly separable input classes.
Frank Rosenblatt, in addition to physically implementing it, also studied a simple algorithm for training.
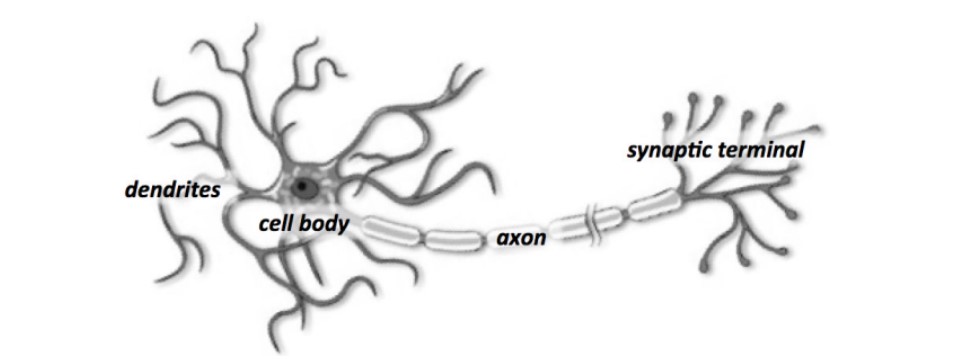
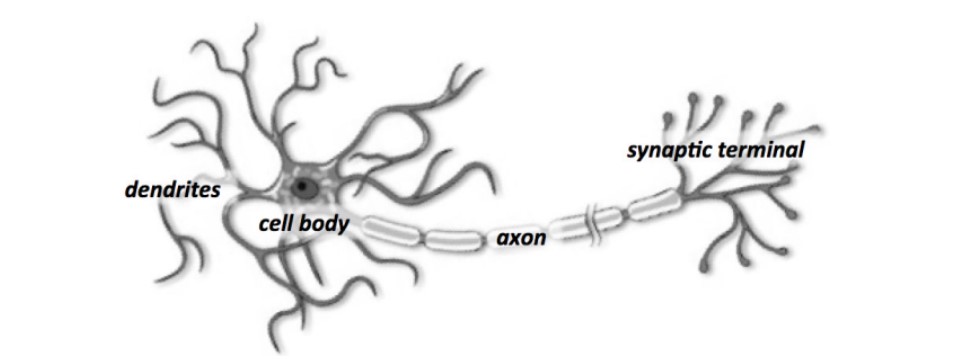
NEURON
HOW HUMAN NEURONS WORK
1) the neuron is optimized to receive information
2) the neuron receives its inputs along dendrites
3) each of these input connections is dynamically strengthened or weakened according to the frequency with which they are used
4) the strength of each connection determines the contribution of the input to the
input to the output of the neuron
5) after being weighted according to the strength of the respective connections, the inputs are summed in the cell body
6) this summation is then transformed into a new signal that is propagated along the axon of the cell and sent to other neurons
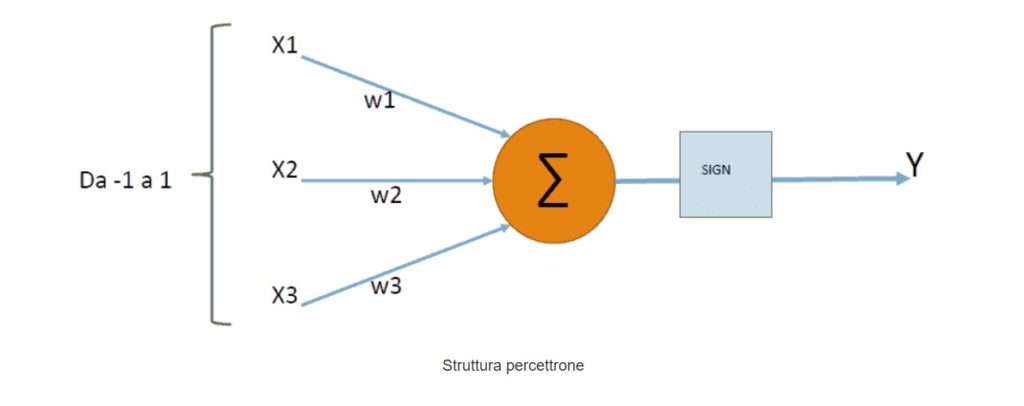
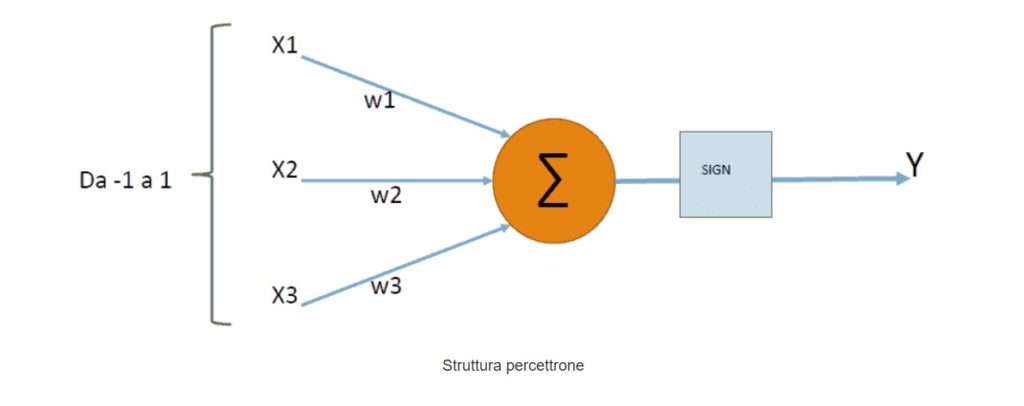
PERCETRONE STRUCTURE
As mentioned above, the structure of the perceptron is very similar to that of a biological neuron, and the various sections are very similar.
We have the dendrites together with the synapses that constitute the input of the neuron.
The nucleus and finally the axons that constitute the signal output from the neuron to go to interact with other neurons.
We then turn to the analysis of the elements that constitute the perceptron.
Similar to the biological neuron, the perceptron consists of :
– At least two or more input connections or synapses
– a calculating core or summing machine
– from an output or also called activation
How it works
I initially assign either zero or a very low infinitesimal value to all the w-weights of the neuron. Then I run the perceptron learning algorithm. I calculate the output y of the neuron based on the current vectors X and W I update the vector of weights (W) of the neuron by the perceptron weight update rule.
Simply put within a Percetron a piece of data, or a string of data, is entered and a “weight” is inserted into the Percetron, which is a form of deformation of the intial data that is processed into its own variant that will have an output data that is relative to the initial information but, different and adherent to the “existence” rules of the initial data.
If you think about it, it is a unique form of creation, very similar to the system by which we process our original reflections or thoughts.
… AND THE RELATIONSHIP WITH TOURISM? THE IMAGE SIMILARITY SEARCH.
Artificial Neurons are the fundamental units of Deep Learning. Not going into a very technical topic, which we will deal with more specifically in the Future, we can define it as follows:
Deep learning is hierarchical machine learning, a segment of the branch of artificial intelligence (AI) that mimics the way humans acquire certain types of knowledge. It is an ad hoc method of machine learning that incorporates artificial neural networks in successive layers to learn from data in an iterative manner.
So, deep learning is a technique for learning by exposing artificial neural networks to large amounts of data in order to learn how to perform assigned tasks.
Deep learning, then, is an important element of data science, which includes statistics and predictive modeling. In fact, it is the learning by which “machines” learn data through the use of algorithms, especially statistical computing.
Deep Learning is applied with excellent results for all those systems or fields that deal with Images or Audio Data.
In Tourism, for example, we at A.I.LoveTourism use reverse image search engines; for example Google for image search . Simply acquire one or more photos and the search engine will bring back photos similar to those introduced, in this way you can find in which part of the World you can find places close by territory to the initial images and guide a territorial promotion marketing in the areas resulting from the similarity search.
This particular Google search works, precisely, through Neural Networks and has a very high search efficiency. The applications are many and often have as their only limitation the imagination of the user
Below, one of our tourism products . It would be interesting to have your opinion on the work we are doing
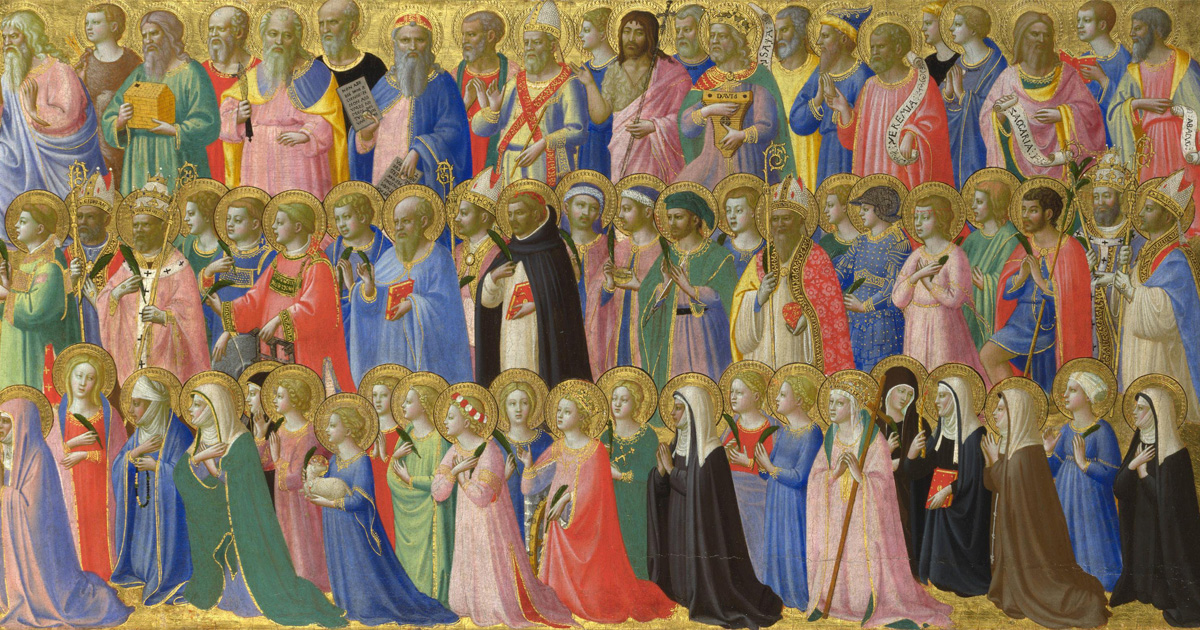
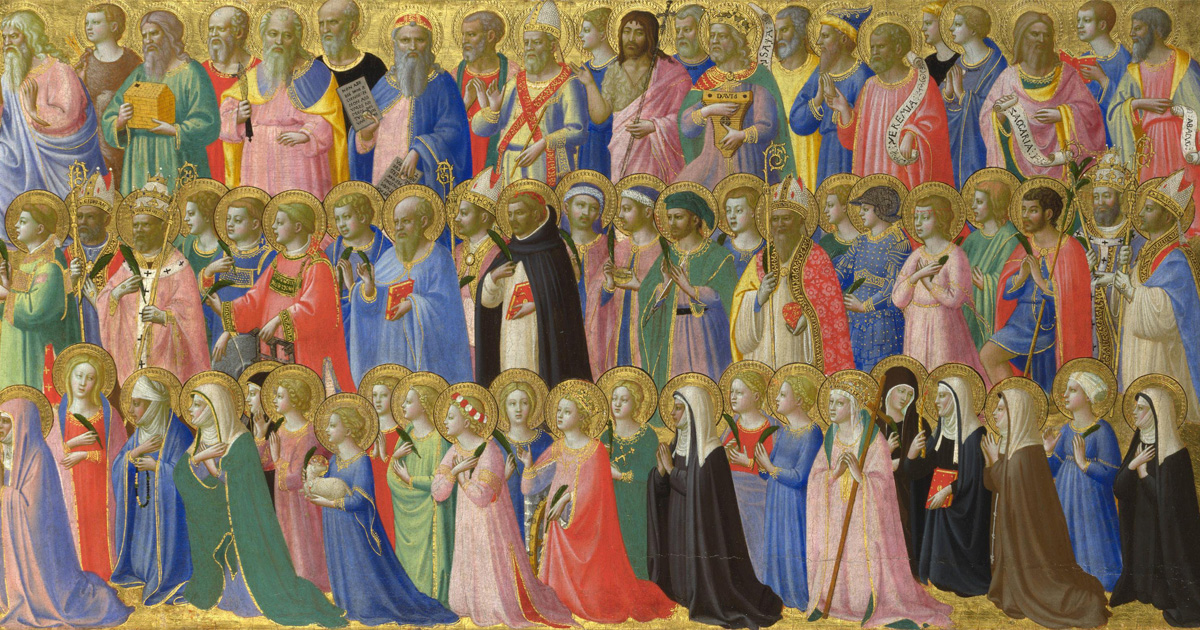
All Saints plus One
One of our Apulia travel packages to discover how the Deities of the 1300s are our recent Superheroes
Contact Us for a data driven trip in Italy
Address
via Ammiraglio Millo, 9 ( Puglia – Italy )
info@ailovetourism.com
Phone
+39 339 5856822